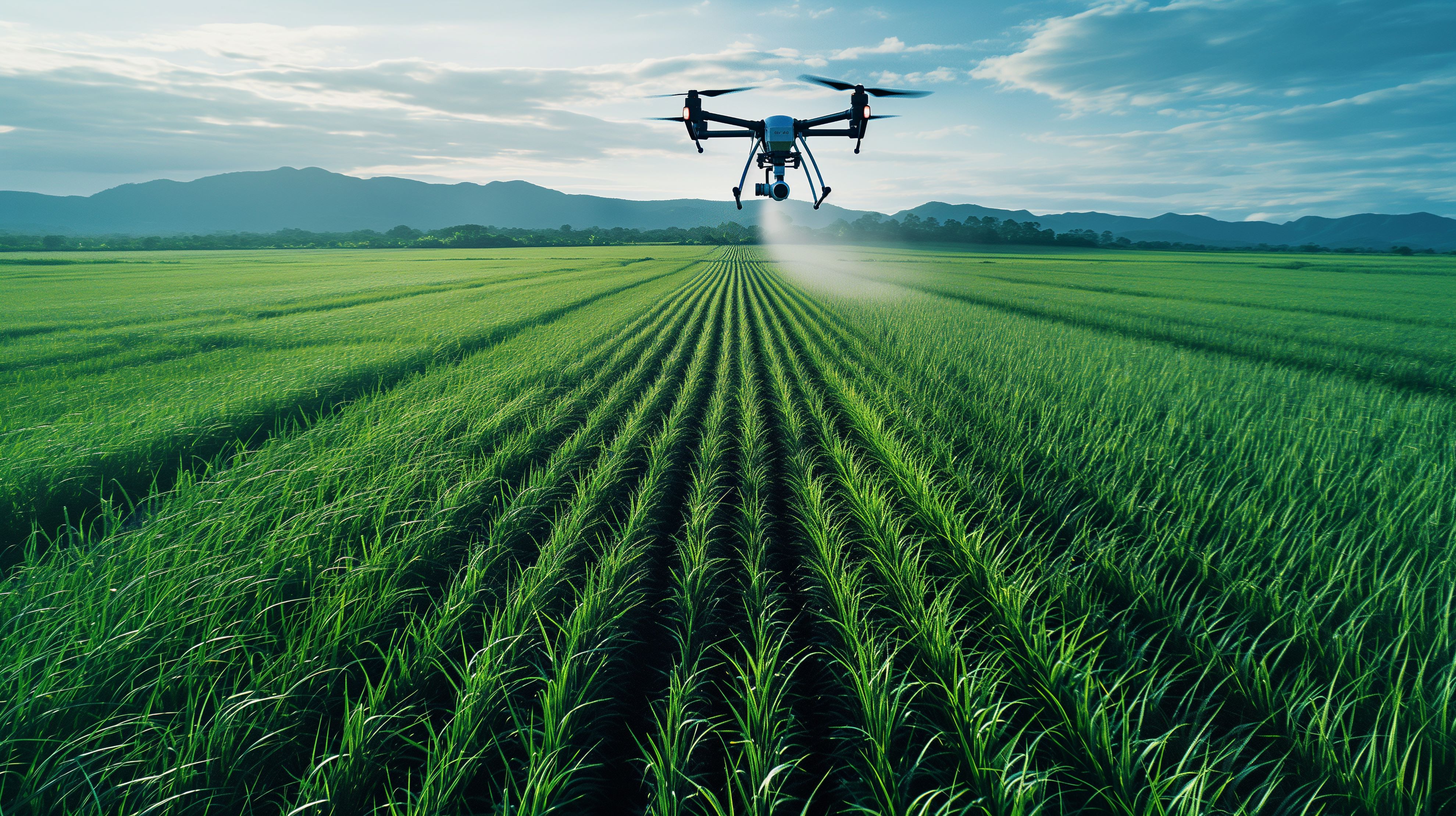
Accurate 3D Reconstruction with Multi-View Imagery: Scalable Solutions for Digital Agriculture
Project Outline
Accurate 3D reconstruction of crops and agricultural environments in digital agriculture brings precision, efficiency, and sustainability to farming practices. By providing detailed and accurate 3D models, it enables better monitoring, resource management, yield prediction, and overall farm management. This supports the move towards smarter, data-driven agricultural practices, ultimately enhancing productivity and sustainability in the agricultural sector.
This project aims to develop accurate, scalable, and efficient 3D reconstruction approaches using multi-view imagery for digital agriculture. Firstly, the research will explore and utilize novel 3D representations, such as neural implicit representation and 3D Gaussian Splatting, which are expected to offer significant improvements in the speed, scalability, and accuracy of 3D reconstruction. The study will evaluate their performance and applicability in large-scale agricultural settings, where fast and accurate reconstruction is crucial.
Secondly, the study seeks to integrate expert prior knowledge of biological structure information, (e.g. functional structural plant models (FSPM)), into the reconstruction framework. By doing so, it will enforce structure-aware reconstruction, ensuring that the reconstructed 3D models accurately reflect the biological and structural characteristics of the plants. This integration will help in providing a more detailed and realistic representation of crops, enabling better monitoring and management in digital agriculture.
Finally, this study will develop methods to account for and mitigate the effects of noisy camera poses, thereby enhancing the robustness and reliability of the 3D reconstruction process. This will ensure that the models are accurate and useful even in less-than-ideal data collection conditions such as using drone to capture image in large scale.
The outcomes of this research are expected to provide promising economic and environmental benefits and lay the groundwork for future innovations in digital agriculture.
What the student will learn from engagement in the project:
Engagement in this project will provide the student with a comprehensive set of skills and knowledge, including:
The student will have advanced understanding of 3D Reconstruction using multi-view images by learning in-depth knowledge of state-of-the-art 3D reconstruction methods and learning how to implement and evaluate the performance of these methods in real-world agricultural settings;
The student will learn to incorporate functional structural plant models (FSPM) into technological frameworks, ensuring that biological and structural characteristics are accurately represented in 3D reconstructions, which can help understand the significance of biological constraints in enhancing the accuracy and utility of digital models in agriculture.
The student will gain practical experience in digital agriculture through hands on experience in collecting and processing agricultural data using drone and other sensor. Further, the student will understand the practical challenges and solutions in implementing advanced digital technologies in agricultural environments.
The student will be engaged in collaborative work with experts at ANU and CSIRO, including crop breeders, biologists, and computer vision experts. They will also have opportunities to directly engage with some of CSIRO’s existing industry partners. These interactions will provide the student with valuable interdisciplinary research experience, and a better understanding of industry to prepare them to tackle real-world problems effectively in their future career.
The student will develop research and communciation skill through comprehensive literature review, experimental design, data analysis, interpretation of results, academic paper writing and participation in conferences and other relevant events and trainings.
Through engagement in this project, the student will emerge with a well-rounded skill set, combining technical expertise with practical experience and research capabilities, positioning them for a successful career in digital agriculture and related fields.
To register an expression of interest, click here. You will need to outline why you have selected the research project and how your skills, experience and/or knowledge meet the project requirements.