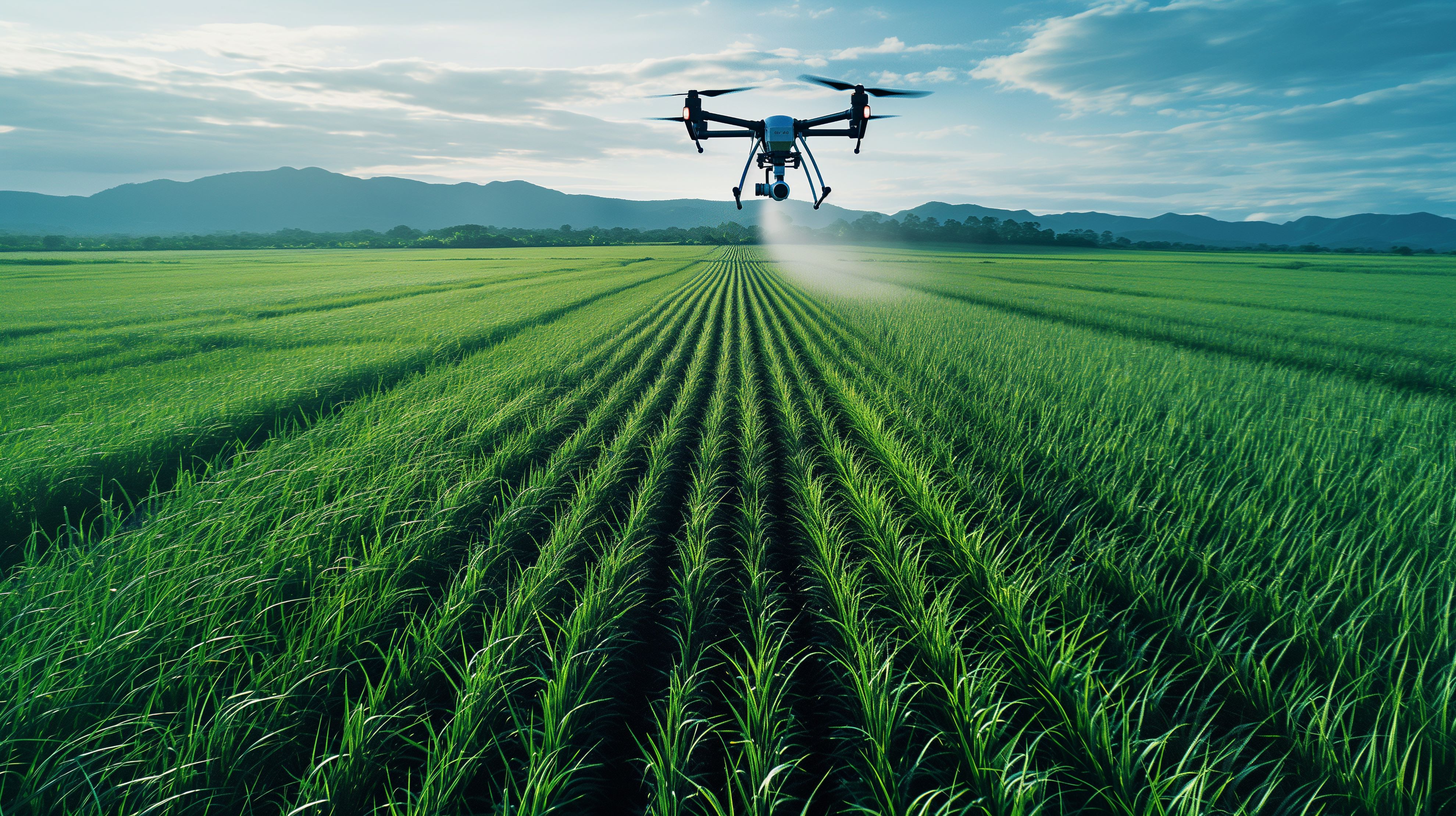
Data-Driven Precision Farming by Multi-Modal Foundational Models and its Application to Plant Photosynthesis
Project Outline
This project aims to empower precision farming with data science and agriculture-specific AI technologies. Specifically, we will build, evaluate and deploy a multi-modal foundational model-based data-driven approach for high-throughput characterisation of photosynthesis in canola leaves and pods.
Canola (Brassica napus L.) is the third most important broadacre crop in Australia, a major edible oilseed globally, and an emerging bio-energy crop. Understanding and enhancing the photosynthetic efficiency of its key organs, i.e., leaves and pods, at different growth stages is important for improving yield and resilience to environmental stresses. Traditional methods of photosynthetic characterisation are often labour-intensive and time-consuming.
We will transform the traditional scientific process of collecting data from field and laboratory experiments into an autonomous, robust and responsible AI solution. Specifically, we will use various data including hyperspectral images and thermal images to capture the underlying phenotypes. The project will be built on Data61’s highly scalable MAGDA platform (https://magda.io/), enabling the traceable and verifiable linking of the multi-modal data from field and laboratory experiments. Using this data, we will train agriculture-specific multi-modal foundational models and design AI agents and Human-AI collaboration tools for autonomous data analytics and scientific discovery.
The data-driven approach, AI models and tools developed in this project will significantly automate the measurement of photosynthesis in canola leaves and pods and will be relevant to other crops by providing rapid, accurate, and high-throughput assessments. We will integrate the new knowledge, models and tools into crop management systems and breeding programs, which will generate broader impact on precision farming.
The PhD candidate will explore the following key research questions through coherent, interdisciplinary research methods:
a) How to design data-centric AI approaches to reliably collect, store and verify high-quality multi-modal sensor data, e.g., hyperspectral imaging spectrometer and thermal camera, to characterise canola leaf and pod photosynthesis accurately?
b) How to effectively learn and predict photosynthesis efficiency/rate change under challenging scenarios such as the range of environmental conditions experienced by the crop at different growth stages where opportunities for data collection are limited?
c) How to ensure the robustness and verifiability of AI systems through effective Human-AI collaboration?
d) How to incorporate data analytic and AI prediction results into existing crop models (e.g., APSIM) to assist in breeding selections?
The student will learn
This team will provide the student with a diverse set of skills and knowledge, including gaining knowledge in plant physiology and data science and AI; developing expertise in planning and conducting experiments, applying statistical methods and machine learning techniques to process multi-modal agriculture data, analysing complex datasets and extract insights from the data; gaining experience in designing, developing and validating AI models and tools, as well as the application of AI technologies to complex crop model development and application.
To register an expression of interest, click here. You will need to outline why you have selected the research project and how your skills, experience and/or knowledge meet the project requirements.