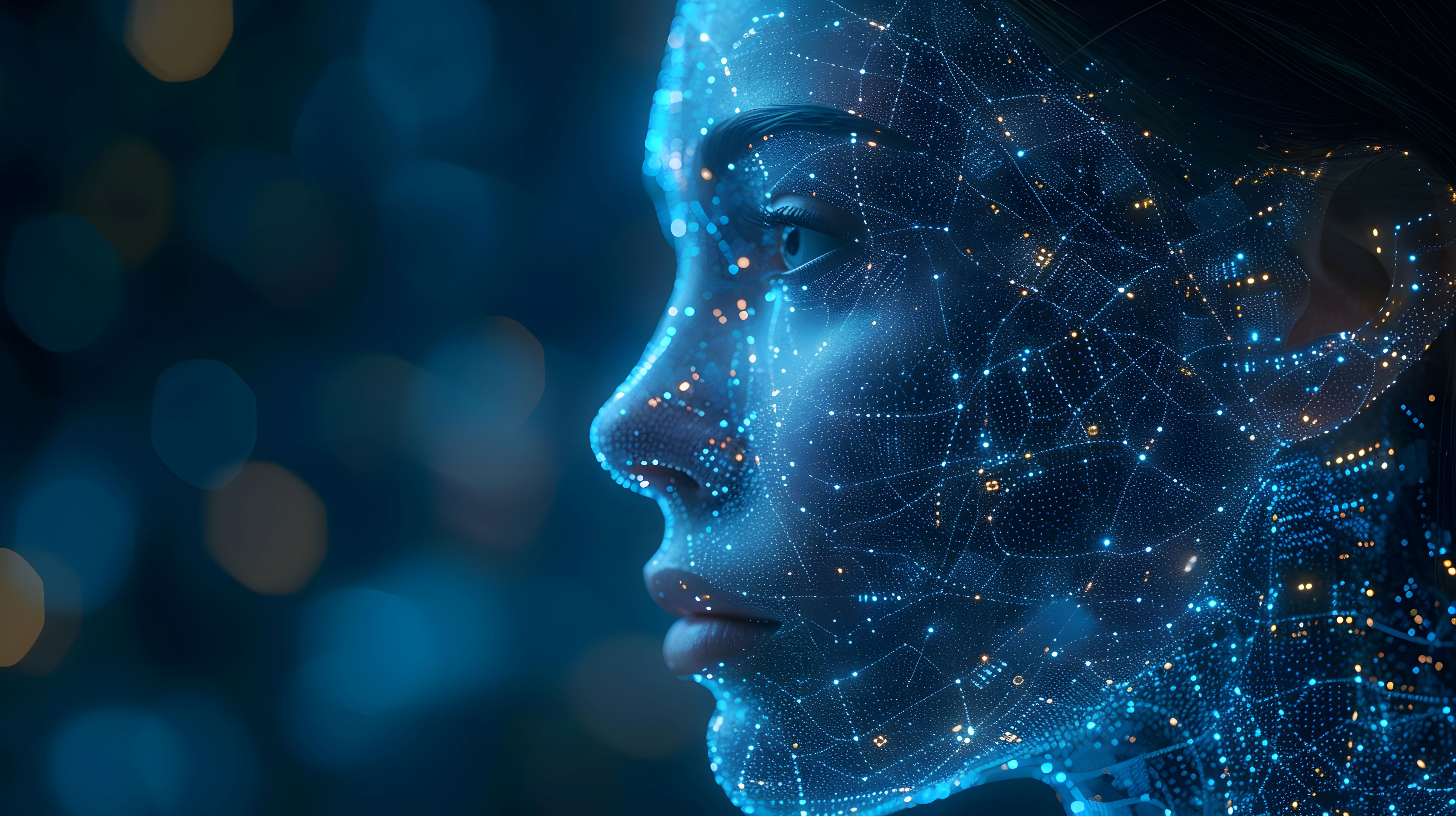
Early Disease and Pest Detection in Agriculture through 3D Computer Vision and Hyperspectral Imaging Techniques
Project outline
This project aims to address the critical issue of crop health management in Australia. With agriculture contributing approximately 3 per cent to Australia’s GDP and employing over 250,000 people, effective disease and pest detection is vital for ensuring food security and economic stability (Australian Bureau of Statistics, 2022). Current estimates suggest that plant diseases and pests cause up to 20-40 per cent yield losses globally, which translates to significant economic impacts (Savary et al., 2019).
This project proposes the development and deployment of advanced 3D computer vision and hyperspectral imaging technologies to detect diseases and pests at early stages. By integrating these cutting-edge technologies, we aim to provide farmers with real-time, precise, and actionable data, significantly improving crop management practices and reducing losses. The project's initial focus will be on detecting diseases in sugarcane, specifically utilising data from the SugarCane Disease project, including hyperspectral datasets for smut and rust diseases. However, the overarching goal is to develop techniques that are applicable to disease detection in a wide range of crops. The project aims to solve fundamental problems of weak signal detection and fine-grain analysis. Building on the existing sugarcane data, the project will also incorporate field trials to validate and refine the techniques for broader agricultural applications The successful implementation of this project could revolutionise agricultural practices, not only in Australia but globally, by enhancing early detection capabilities and minimising crop losses due to diseases and pests.
3D computer vision technology can play a vital role in this project by creating detailed models of crops and their environments, enabling precise monitoring of plant growth and early identification of abnormalities. This technology can detect subtle changes in plant structure that are indicative of disease or pest infestation, which might be missed by traditional visual inspections. By capturing spatial information about plant canopies and leaves, 3D computer vision can help identify patterns and anomalies that signify the presence of pests or diseases, even before visible symptoms appear.
Hyperspectral imaging, on the other hand, captures a wide spectrum of light beyond the visible range, providing detailed information about the biochemical composition of plants. This technology can detect specific wavelengths of light that correlate with plant stress, disease, or pest infestation. For example, changes in chlorophyll concentration, water content, or the presence of specific pathogens can be identified through hyperspectral signatures. By analysing these spectral data, hyperspectral imaging can diagnose plant health issues at a molecular level, offering an unparalleled level of precision.
By integrating these cutting-edge technologies, we aim to provide farmers with real-time, precise, and actionable data, significantly improving crop management practices and reducing losses. The project will involve extensive field trials, leveraging Australia’s diverse agricultural landscapes to ensure the solution's robustness and scalability. The combination of 3D computer vision and hyperspectral imaging will create a comprehensive monitoring system that enhances early detection capabilities, allowing for timely interventions and reducing the reliance on chemical treatments. The successful implementation of this project could revolutionize agricultural practices, not only in Australia but globally, by enhancing early detection capabilities and minimising crop losses due to diseases and pests.
What the student will learn from engagement in the project:
Engaging in the multidisciplinary project will provide a PhD student with a comprehensive learning experience. The student will master 3D computer vision, creating detailed models of crops to detect subtle structural changes indicative of disease or pest infestation. They will gain expertise in hyperspectral imaging, capturing and analyzing spectral data to identify plant health indicators like chlorophyll concentration and water content. Handling large datasets, they will focus on preprocessing, cleaning, and annotating data, and develop machine learning models for pattern recognition and anomaly detection, addressing fundamental AI problems of weak signal detection and fine-grain analysis.
The project will enhance the student’s skills in integrating 3D computer vision and hyperspectral imaging into a cohesive system, developing Artificial Intelligence (AI) solutions for disease and pest detection. Working with experts in agronomy, computer science, and engineering will improve their interdisciplinary collaboration and communication skills. They will design and conduct field trials, ensuring the scalability and robustness of the developed techniques for diverse agricultural settings.
Through impactful research, the student will contribute to revolutionising agricultural practices by enhancing early detection capabilities and minimising crop losses. Opportunities to publish research findings in high-impact journals and present at international conferences will further their academic and professional development. By the end of the project, the PhD student will be well-equipped with the technical skills, knowledge, and experience necessary for a career in academia or industry, particularly in agricultural technology, computer vision, and hyperspectral imaging.
To register an expression of interest, click here. You will need to outline why you have selected the research project and how your skills, experience and/or knowledge meet the project requirements.