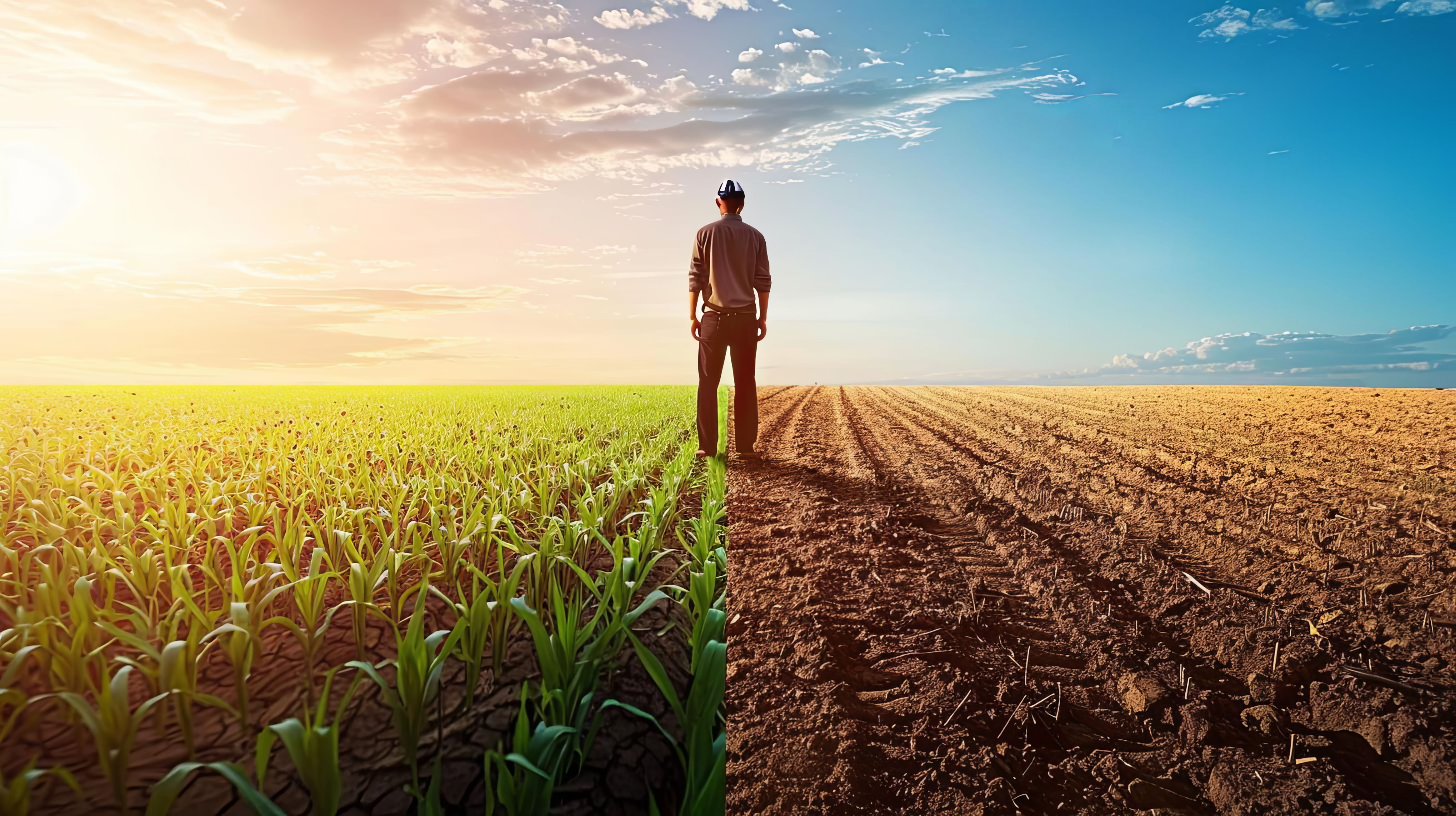
Sensing the next step change in water productivity in dryland agriculture
Project Outline
Better knowledge of soil water content at paddock and farm scale underpins improvements to almost all decisions facing agriculture in an increasingly variable climate. If, when and what crops to sow, how much fertiliser or other inputs to use, whether to cut crops for hay or grow on for grain in droughts and even yield predictions for marketing purposes are all decisions that can be influenced by a more accurate measurement of the water available to plants in the soil. Historically, soil water availability was estimated from rainfall data using simple calculators and local knowledge of the soil, but new soil water sensing technology is making it increasingly possible to measure soil water in real time. Wireless soil water sensors that can monitor soil water in real time to 2m depth are now a reality, but the value of that information in the context of variable seasonal outlooks is uncertain. Knowing how much water is available to plants in the soil, together with seasonal forecasts could be transformational in de-risking management and input decisions - key to improving productivity and water-use efficiency of our dryland systems and reducing environmental impact on soils, water, and landscapes.
The PhD candidate will use farming systems analysis and modelling to determine the benefits of real-time knowledge of plant-available water in on-farm decision-making. The candidate will develop an in-depth understanding of soil water use in cropping systems, and the impact of management decisions on water-use efficiency, drawing on data from the CSIRO Boorowa Agricultural Research Station (BARS) and other long-term cropping trials for verification and to augment the simulated data.
The student will learn
1) Training in agronomy, simulation and associated data analysis and machine learning to further explore model output. A background including coding in either R or Python would be desirable.
2) General field and laboratory work methods including soil sampling, soil physical and chemical analyses, and related analysis of data.
3) An in-depth understanding of the crop water balance, crop physiological responses to water deficit and the interactions in farming systems that lead to improved water-use efficiency.
4) Exposure to, and understanding of, the latest soil and crop sensing techniques deployed in digital agriculture, including at commercial scale.
5) Transferable core scientific skills including data management, analysis, presentations, paper writing, and peer review, as well as working in a multi-disciplinary team.
To register an expression of interest, click here. You will need to outline why you have selected the research project and how your skills, experience and/or knowledge meet the project requirements.